When discussing conversion rate optimisation (CRO), one concept often causes confusion: statistical significance. What does it mean? Do you need to study statistics now? Don’t worry. We’ll explain it in simple terms, and no, you won’t need to study statistics.
In this blog, we’ll explore the concepts of statistical significance and confidence levels. We’ll explain why statistical significance is crucial in CRO and compare two statistical methods: the Frequentist and the Bayesian.
What is Statistical Significance?
Statistical significance refers to the likelihood that a result from testing or experimentation is caused by something other than chance. In CRO, after formulating a hypothesis, statistical significance helps prove the winner.
Frequentist vs. Bayesian
There are two approaches to proving statistical significance: the Frequentist and the Bayesian models. There’s ongoing debate in the CRO world about which approach is best. The Bayesian model is more intuitive and based on random variables, while the Frequentist model is more conservative and based on fixed variables. Both methods are valid, but they differ in their application and interpretation.
The Frequentist Approach
In the Frequentist approach, you start with the assumption that there’s no difference between A (control) and B (variant), known as the null hypothesis. You analyse results from a sample of your website audience during the experiment.
Results depend only on the data captured during the experiment. A challenge with Frequentist statistics is that you have only two possible outcomes: your experiment is either a winner or not. You either prove the null hypothesis right or fail to prove it, with no room for interpretation.
The level of statistical significance, or p-value, ranges between 0 and 1. By convention, if the p-value is > 0.05, the null hypothesis cannot be rejected, meaning the difference between A and B could be due to chance. A p-value of < 0.05 means the null hypothesis can be rejected, indicating a statistically significant difference.
Translating this into percentages: to declare a winner, the test results need to reach a confidence level of at least 95%.
The confidence level indicates how certain you can be. A 0% confidence level means no faith in replicating the results. A >99% confidence level (100% doesn’t exist in statistics) means near certainty in replicating the results.
If you can’t identify a significant winner, don’t discard your test right away. You might need more conversions or a higher sample size. AB Tasty has an easy calculator to determine your ideal sample size for statistically significant results. Also, it’s recommended to run the test for at least two weeks.
The Bayesian Approach
The Bayesian method is more intuitive, based on the “chance (of the variant) to beat control.” Bayesian inference provides the probability of B being better (or worse) than A, and by how much. The results from an experiment inform possible future outcomes.
Though the calculations can be complex, the Bayesian approach can be simplified to probabilities. For instance, “The variant is better than control with a 70% probability” or “The variant has a 70% chance of beating the control.”
Here’s a simplified explanation:
Posterior: Result from analysis (prior and evidence)
Likelihood: Observed data
Prior: Data from previous experiments
Evidence: Results of the current experiment
The Bayesian approach offers a 0 to 100% probability of the variant performing better than the control. To prove a hypothesis, anything equal to or above 80% for a positive uplift or equal to or below 20% for a negative uplift has a strong probability of beating the control. For better accuracy, a larger sample size and higher probability are preferable.
An advantage of the Bayesian approach is its effectiveness with smaller sample sizes, allowing faster decisions. However, the results don’t have the same degree of statistical significance as the Frequentist approach. While a statistician might view Bayesian results as an educated guess, in a business scenario, this can be sufficient.
Conclusion
You don’t need to pick a side between the Frequentist and Bayesian approaches. The key is to understand the results of your experiments using your chosen CRO platform. Whether you opt for a more conservative or intuitive approach, grasping the basics of statistical significance will help you make informed decisions and improve your CRO efforts.
Share this article
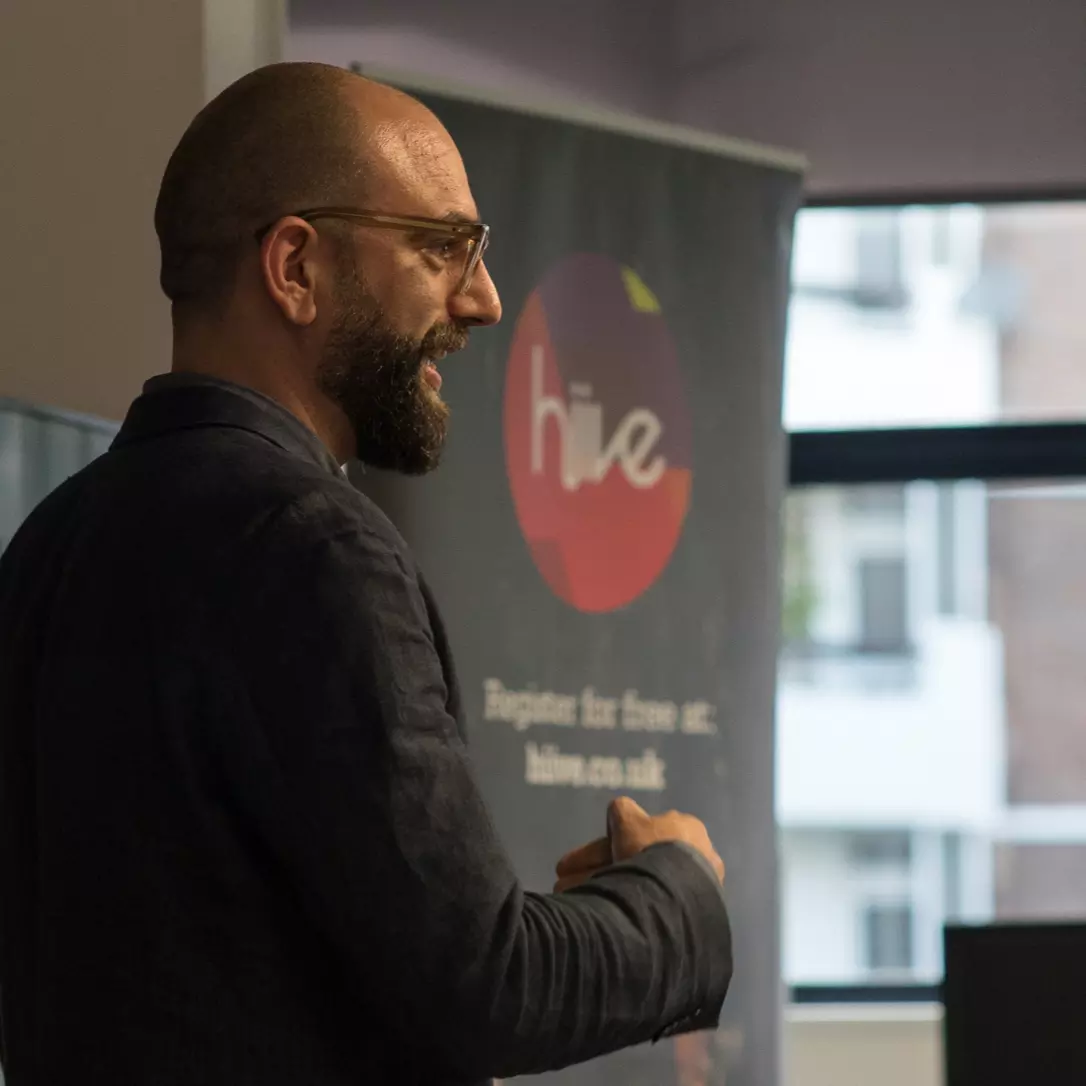
Contact us to discuss how experience-led tech can make your digital work harder
Get in TouchRelated articles
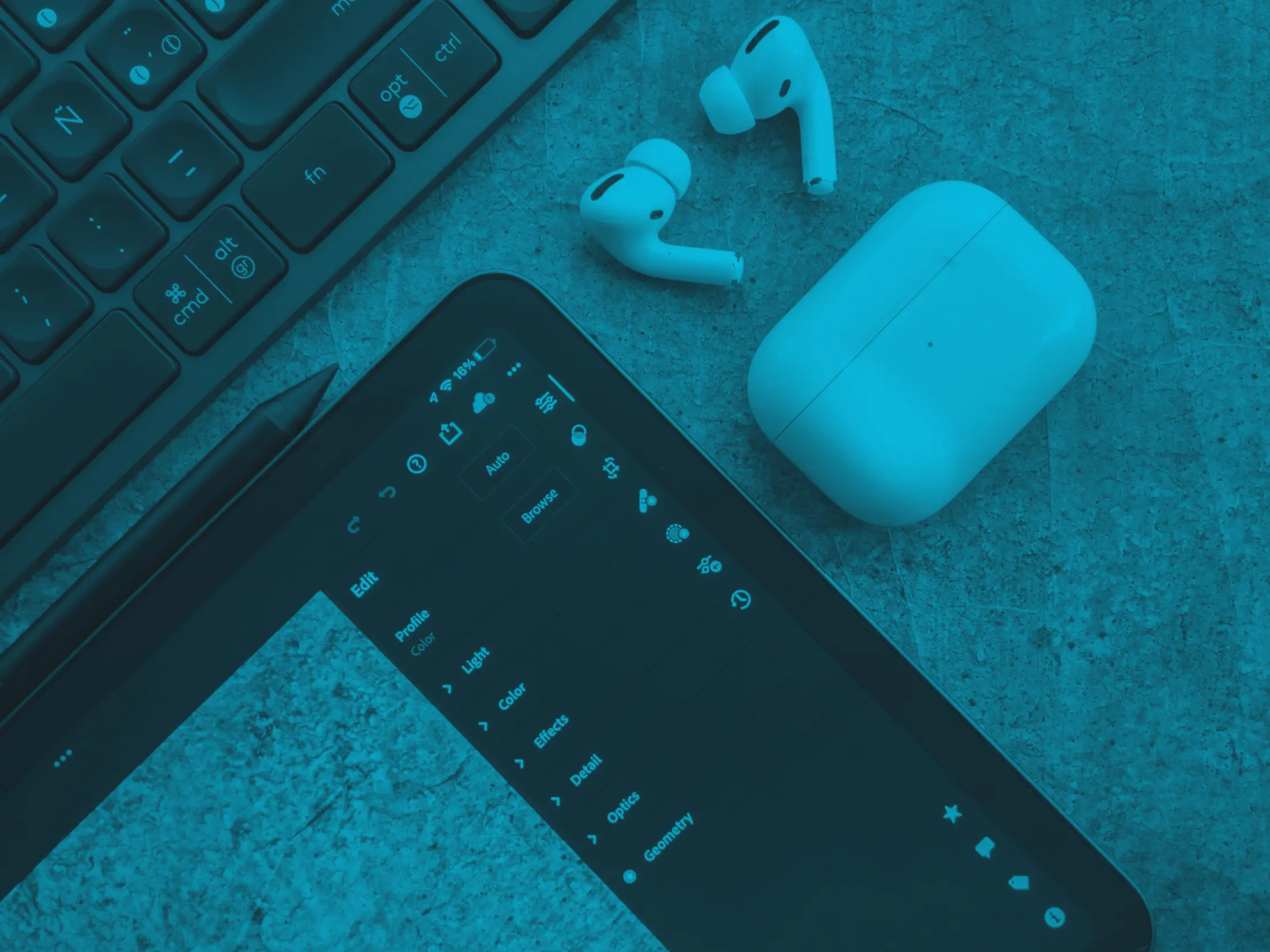
Blog
6 Things Successful Freelancers Don’t Say
By Cohaesus. Apr 29, 2015
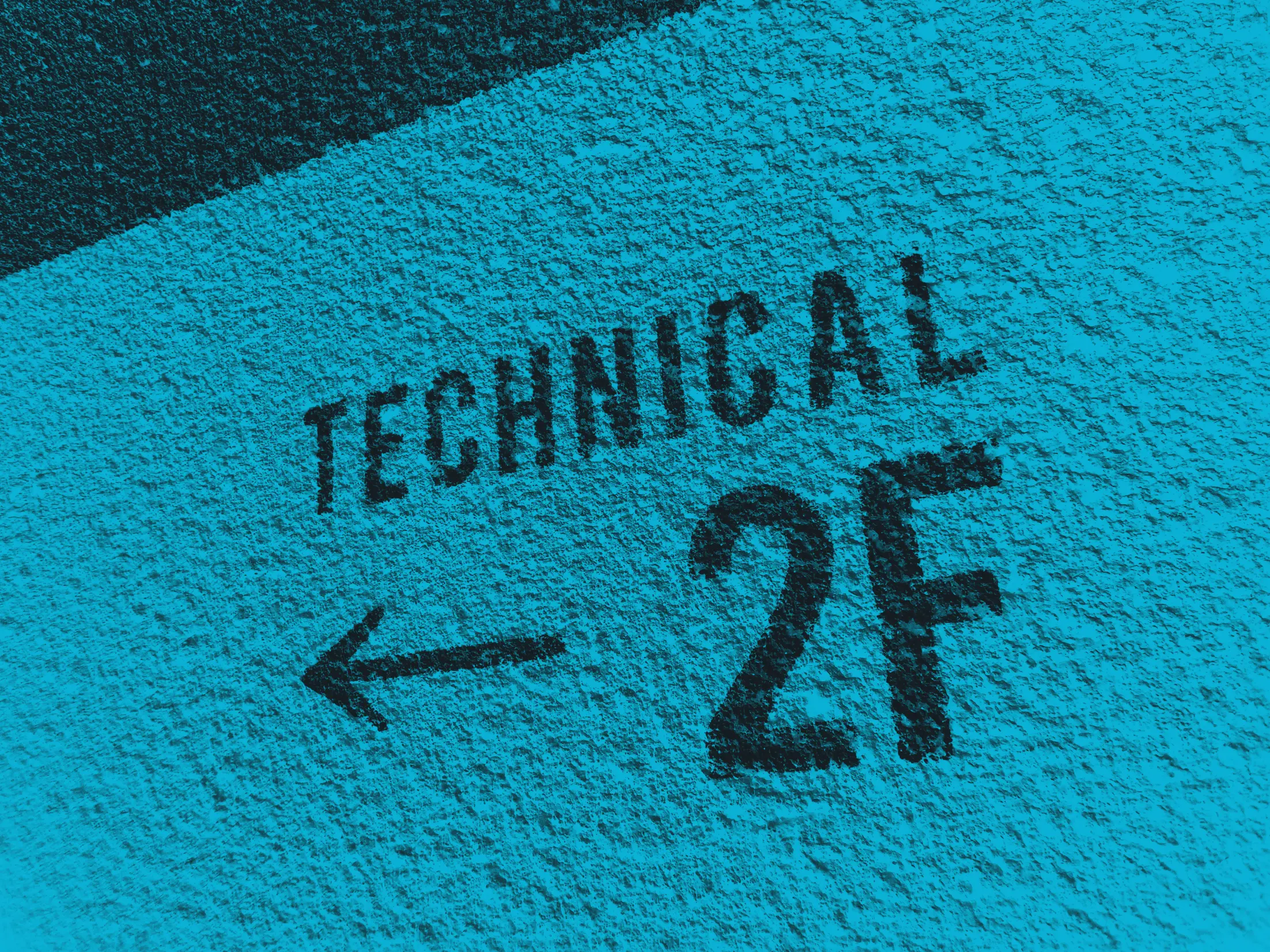
Blog
5 questions to ask before choosing your technical partner
By Cohaesus. Apr 05, 2016
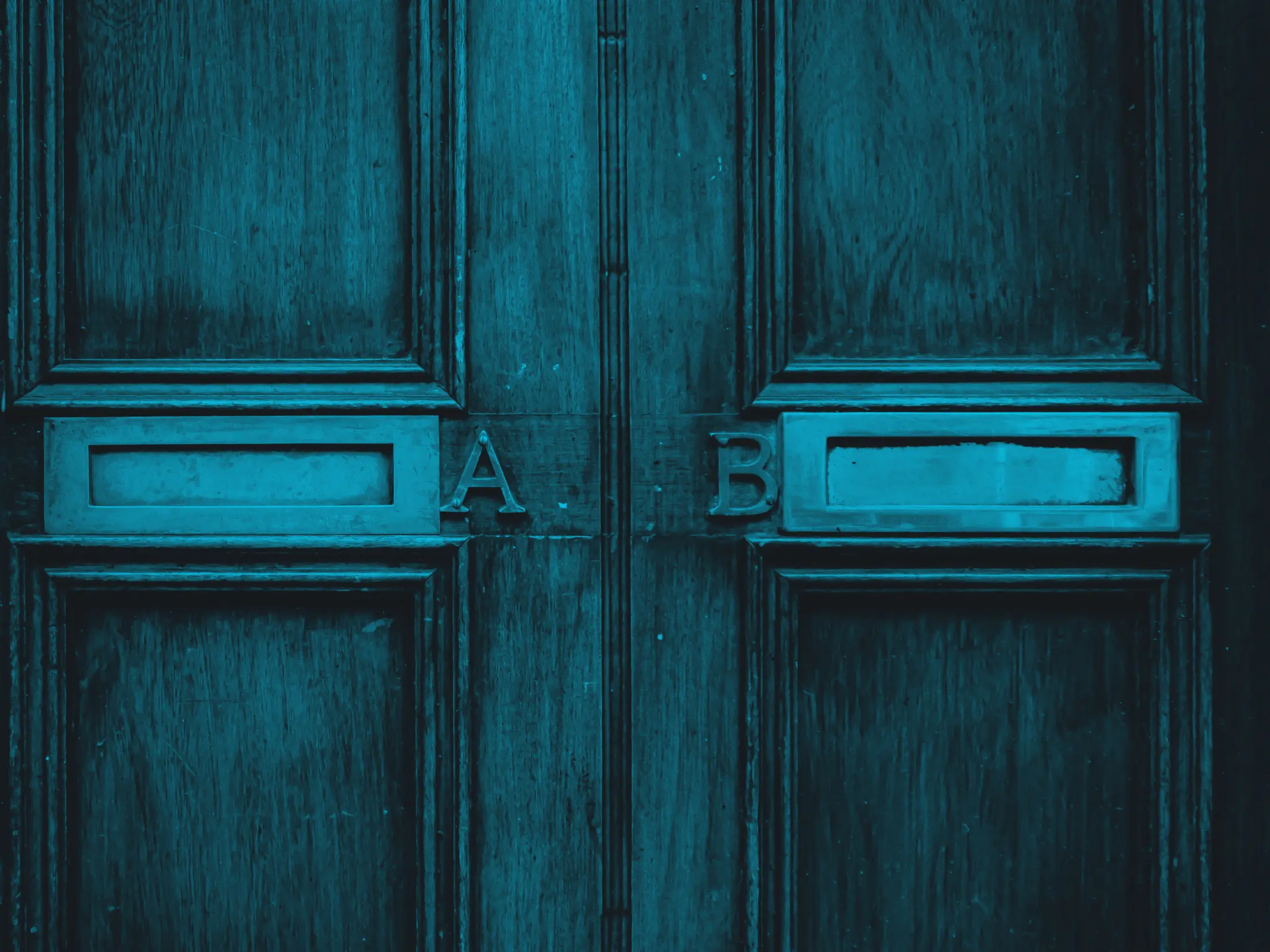
Blog
7 Simple A/B Test Ideas to Start With
By Cohaesus. Jan 16, 2025
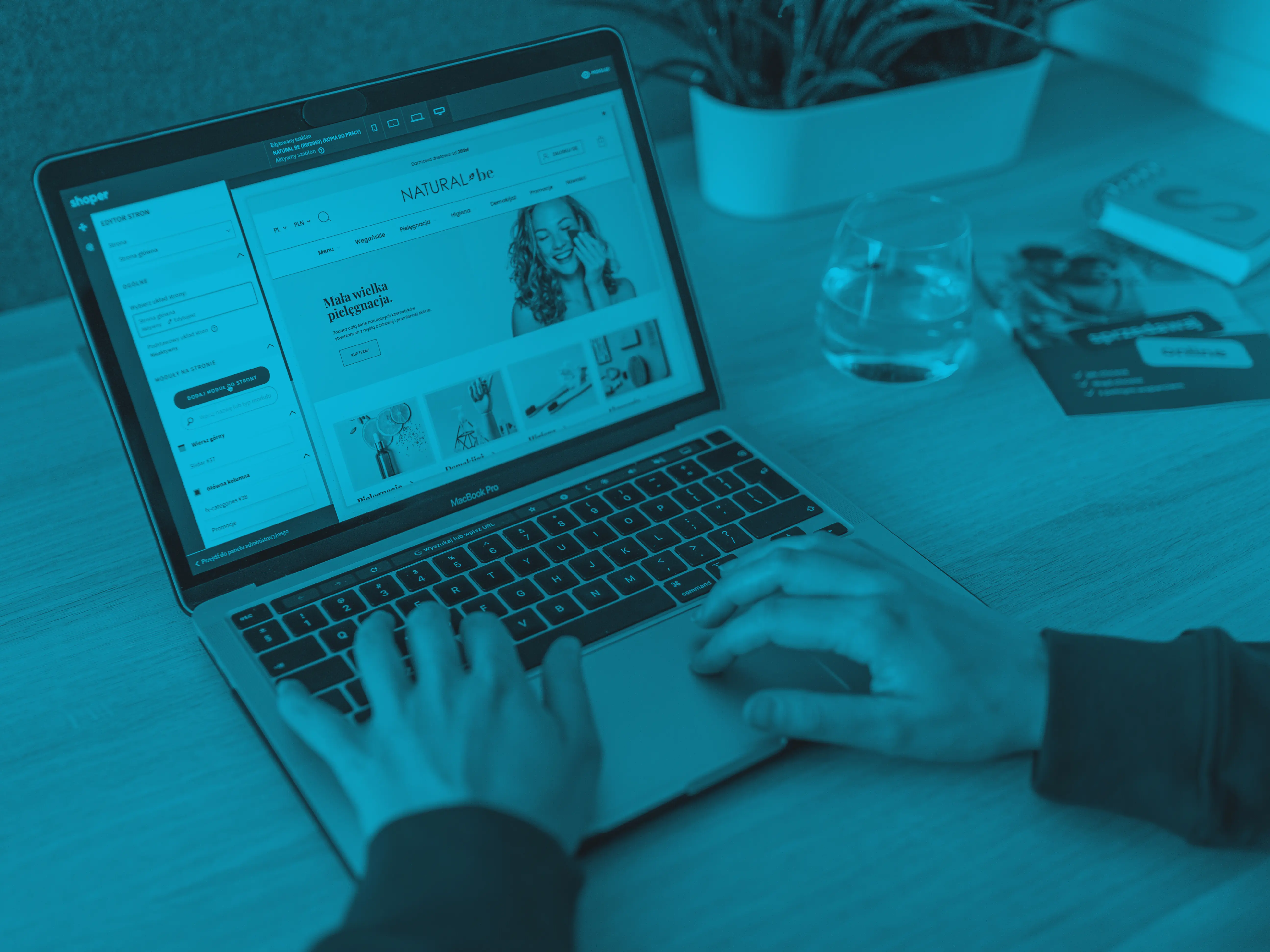
Blog
The Cohaesus Guide to Replatforming Your E-commerce Solution
By Cohaesus. Jan 16, 2025